Feeding the world with AI

Categories: Energy and sustainability Technology
When we hear about food waste, we tend to think of wastage at the consumer side of things – the bag of half-eaten salad mix you guiltily throw out every week, the enormous meal at a restaurant you couldn’t finish, or your parents sternly reminding you of the ‘starving children around the world’ as you pick at your peas. Food loss and wastage, however, is a pervasive issue at all stages along the food supply chain from production and storage through to transport and consumption.
The Food and Agriculture Organization of the United Nations (FAO) claims that one-third of food produced for human consumption is lost or wasted globally – equal to around 1.3 billion tonnes annually.
While consumer-side efforts have been launched in recent years to combat this issue (such as ‘ugly’ fruit and vegetable campaigns, and apps that let consumers buy cheap food from cafes before it gets binned), there’s an opportunity to combat the issue on the production-side by harnessing AI and machine learning (ML) technology.
Using AI and Machine Learning in Aquaculture
Aquaculture is a particularly complex subset of the food production ecosystem, from both a technical and political perspective. It’s highly regulated and highly susceptible to the elements. It’s a high-risk crop that generally offers high-value. For example, one of the core issues for oyster growers is harvest area closures. As filter feeders – animals that feed by straining suspended matter and food particles from water – oysters are highly susceptible to contamination from runoff.
To prevent this contamination, harvest areas typically close when rain is forecast in the area. While a necessary exercise to ensure food safety, the problem with closing these areas is the resulting impact on how many oysters that growers can harvest. On top of this, if a harvest area is closed but it doesn’t then rain (or, conversely, rains too heavily), then over time growers end up harvesting fewer oysters and losing money – a costly problem.
A possible solution
We developed Sensing+ for Aquaculture to leverage AI and ML in order to solve problems – like the one described above – currently facing the Australian aquaculture industry. By combining IoT technology with advanced AI and analytics, oyster growers in Australia can make more accurate predictions. Real-time sensors collect data on the health of the water in the harvest area, as well as atmospheric and weather conditions; we then use artificial intelligence to predict closures with over 95% accuracy, three days in advance. In practice, the technology has produced a 30% decline in unnecessary harvest closures – reducing risk in the oyster supply chain.
Using AI and Machine Learning in Agriculture
The impact that AI can – and has – have on aquaculture is immense, but the reality is that only a fraction of the world’s daily consumed calories come from seafood: the bulk of our caloric intake comes from plant sources such as grains, fruit, and vegetables. The obvious question, therefore, was to see whether we could use AI to produce a similar impact on how we produce food crops.
In this endeavour, we developed Sensing+ for Agriculture, which uses on-farm sensors to collect data on growing conditions, such as wind speed and direction, rainfall, relative humidity, soil moisture, temperature, and light radiation. Using artificial intelligence and predictive models, we then convert this data into a seven-day forecast. The more data our sensors collect, the more our data analytics platform learns – which means that as time goes on, predictions get more and more accurate. And, with more accurate predictions, growers can plan on-farm activities with greater confidence, and act before a catastrophic event – such as frost – occurs.
As with the aquaculture solution, accurately predicting weather conditions impacts not only agricultural crop growers’ bottom line, but also the size and quality of their harvests. By optimising water use in intensively-irrigated crops, growers can maintain optimal production and yield. Ultimately, this means a significant increase in food production, and better, more efficient use of precious and finite resources like water.
What next?
To continue feeding a growing global population during a period of unprecedented resource scarcity, we need to look at ways to optimise every stage of the supply chain. It’s not just about increasing production, contrary to popular opinion. It’s also about maximising efficiencies and minimising waste at every stage – from processing and transport to storage and consumption.
We’re already using AI and ML to help reduce waste at the production side, but there are additional opportunities for these highly-localised predictions to impact along the supply chain. Imagine, for example, the impact of knowing the exact date a harvest is ready, and the exact quantity. Armed with that knowledge, crops can be harvested at the point it has the best shelf life, distributors can arrange for prompt storage and refrigeration, and retail networks can ensure adequate supply to the markets where this product will sell best.
This is just the beginning of AI and data science's ever-increasing impact on how we will grow, transport, distribute, and consume food across the world. The ultimate goal has always been to feed the world without destroying the planet, and by harnessing advanced data analytics and artificial intelligence – we’re starting to do just that.
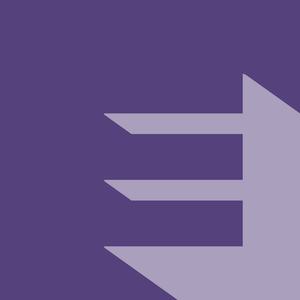