How AI and automation will shape the future of agriculture

Categories: Energy and sustainability Technology
The agriculture industry is fundamental to securing a sustainable future for humanity. Few other sectors have such enormous potential to benefit the planet in the face of population increase, resource depletion, and climate change. For some observers, the focus on agriculture pertains to the simple concern of feeding 9.8 billion people from a static (or worse: declining) arable land base. For others, the focus lies more on the quality of our soils, watercourses and ecosystems, and their ability to support life or sequester carbon. Regardless, the basic mathematical realities of our finite landmass and the competing demands upon it – rapidly changing weather patterns and increasing rates of consumption – lay bare a stark challenge: producing more food from less land, with less waste, lower inputs, and lower environmental burden. According to estimates, we need to produce as much food in the next four decades as we have produced, so far, in the entire history of agriculture - some 10,000 years.
Increasing demands vs. scarcity of labour
Perversely, despite our growing population we need to increase productivity with less labour. Around the world, the average age of a farmer is rising and people continue to leave the profession more quickly than others join it. In every geography, talk of how difficult it is to attract, recruit, train, and retain workers is pervasive. As the workforce dwindles, growers must either find methods to automate or risk being unable to continue.
These driving principles behind today’s agritech industry are the reason why investment is pouring into synthetic biology, data science, artificial intelligence (AI), and robotics – helping to improve all elements of food production.
Delivering individual care on an industrial scale
Such has been the recurring pattern in agriculture through the ages. Every innovation – from the plough to the combine harvester – has seen productivity increase and labour decrease. Machines have grown from the scale of a man, or an ox, to behemoths bigger than tennis courts capable of production rates that exceed an army of human workers. Now, though, we might see this trend reverse.
For some crops, the emphasis is less on the consolidation of a prairie-full of vegetation into endless production lines; rather, it’s on consideration of the individual plants. The emergence of robotics and artificial intelligence allows growers to care for plants as a gardener would – affording each one a level of care and diagnostic support that is impossible for a lone human farmer surveying crops from a passing tractor.
The tip of the spear – high risk, high reward crops
These trends are particularly evident where the profit margins can support the costs of innovative product development, and where the ‘perishability’ of the crop is high. These areas – used to grow tomatoes, berries, and salad vegetables – highlight the benefits of controlling the growing environment and monitoring the health of individual plants. Innovations in indoor farming, using closed-loop control of lighting, water and plant nutrition, have driven improvements in productivity that are rippling down to lower-price, commodity crops. Machine vision systems use multispectral imaging – imaging that records data at several targeted frequencies along the electromagnetic spectrum – to monitor the photosynthetic health of plants and adjust the nutrition accordingly. Current research aims to apply these techniques to diagnose pests and perform treatments, removing the need to preventatively spray expensive and noxious chemicals over crops.
Creating a more predictable future
The benefits extend beyond increasing production in kilos and tonnes – they can, for example, increase the profitability of the farm. Chemicals are expensive, so minimising their use is an obvious cost benefit. More than this, the prices that growers can secure in competitive markets are a function of yield – as the harvest of commodity crops increases, their price decreases, as all farmers achieve bumper levels of productivity. When the harvest is poor, prices rise accordingly. To deal with this uncertainty, traders and growers typically hedge, selling futures on a portion of their harvest. Under these trading rules, farmers relinquish some of their profits in favour of certainty, and the commodity traders and retailers consolidate their risks across multiple markets. If a farmer can accurately predict the exact yield of a crop a month before harvest, then they can secure a price for their produce at a level that radically shifts the profitability of the enterprise – sometimes by a factor of two or even three.
To unlock these opportunities, we need to collect and analyse datasets that comprise a myriad of measurements. These measurements could include the size of every lettuce head in a field, or detail the observed growth progression, colour, and appearance of every apple on every tree, in an orchard spanning hundreds or even thousands of hectares.
A new seed for data-driven agriculture
Providing growers with such precise information throughout the growing season can only be achieved with automated, large-scale data collection. Large-scale monitoring approaches have been made using airborne drones, but these can’t capture information from beneath the crop canopy. What’s more, ground-based monitoring has been, thus far, limited by the need for additional infrastructure. Reliable data capture is one of the challenges that Cambridge Consultants have focused on over the last twelve months.
In March, we announced Mamut: an autonomous robot designed to explore crop fields, capturing data on the health and yield of individual plants, on a massive scale. By automating data capture, Mamut could enable growers to predict and optimize yields – giving them regular, precise, and actionable information on their crops. To capture this volume of data, the surveying needs to be fully autonomous; this was the driving principle behind Mamut, which maps and navigates its surroundings using a stereo camera, LIDAR, and onboard AI. While travelling through the rows of a field, orchard, or vineyard, its cameras capture detailed crop data at the plant level, enabling accurate predictions of yield and crop health. Such a tool allows outdoor growers of lower-priced produce to benefit from the typically-indoor techniques – seen in sophisticated greenhouses or vertical farms – driving recent improvements in plant care.
Mamut
Mamut is a practical application of AI that meets a real and pressing need – particularly for growers of speciality crops, where failure carries a high cost. AI systems can already understand crop conditions and yield predictions and enable weed identification, but Mamut can collect valuable and granular data below the canopy, where drones can’t see. This data allows farmers to treat each plant individually while operating at scale – optimising yields and producing more with less.

From the road to the field
We developed Mamut’s ability to react and learn from unstructured routes in real time in navigation trials, taking it through the twists and turns of both a 12-acre maize maze and an orchard. We needed to apply techniques that are typically central to the development of autonomous vehicles but, in the process, we encountered challenges that are specific to agritech.
For example, while its speed is lower than autonomous road vehicles’, the environment Mamut faces is less structured. Computation requirements are therefore higher; it isn’t possible to rely solely on map data, and the input from sensors must be treated with scepticism and interrogated with a value-judgement. The robot needs to know whether to push through a clump of grass or interpret it as an impassable barrier.
On top of this, data from the cameras can be degraded by raindrops on the lenses, or the presence of dust in the air. The control system needs to understand whether it can trust its sensor inputs, so we are experimenting with ways to retrieve data from damaged or degraded sources. These assessments can’t be coded using simple thresholds; they must be learned through reinforcement, similarly to how a toddler would first learn to navigate through the same space. Such developments advance the fields of robotics and artificial intelligence as much as they improve the productivity, efficiency, and sustainability of the industries in which we apply them.
Looking ahead
Developing Mamut has been an enthralling technical challenge, and we believe that AI-powered robots could play at least a small part in improving the efficiency of future farming. But no single invention will sufficiently solve the crisis currently facing humanity.
The coming decades will see pressures on water and land use become ever more acute, and we must use all the technological tools at our disposal to roughly double food production, from about half the land base, with half the current input energy. We need to build systems that can adapt to changing weather patterns and deliver food without waste to consumers. We need food sources to be closer to the point of consumption, and transportation to occur through more energy-efficient supply chains. From a technology standpoint, at least, this is all possible. Agriculture is pivotal to a sustainable future for humanity, but agritech will help us to secure it.
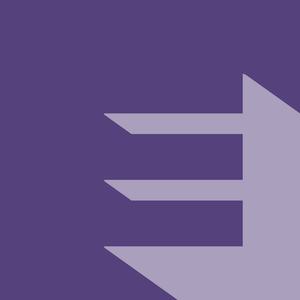
More on the author, Nathan Wrench
Head of sustainability projects at Cambridge Consultants.